Uncover the Magic of AI: A Beginner's Guide to Forecasting with Real-World Use Cases
- SH
- Apr 7
- 4 min read
Artificial Intelligence (AI) is not just a trend; it's reshaping how industries function today. From improving efficiency to making better decisions, AI is changing the game. One of its most practical and impactful uses is forecasting. By leveraging AI to analyze big data, organizations can predict various outcomes such as sales trends, customer demand, and inventory needs. In this guide, we will examine the challenges AI forecasting addresses, the advantages it provides, the tools you can utilize, and the essential skills required for effective implementation.
Challenges Solved by AI Forecasting
Traditional forecasting methods often struggle to adapt to fast-changing market conditions. Static models rely on historical data, which may not reflect current consumer behavior or market dynamics. This gap can result in wasted resources and lost opportunities, putting businesses at a disadvantage.
AI forecasting tackles these issues by learning from fresh data and continuously updating its predictions. For example, a retail company that noticed a sales decline last year might have used outdated methods that could not respond to changing shopping patterns. With AI, they can keep track of consumer preferences in real-time, allowing them to adjust their inventory and marketing strategies quickly.
Benefits of AI Forecasting
Using AI in forecasting comes with numerous benefits:
Faster and More Accurate Predictions: AI algorithms can quickly analyze massive amounts of data. For example, they can process information from thousands of transactions in seconds, providing timely insights. This swift analysis allows managers to make informed decisions swiftly, often cutting down decision-making time by up to 50%.
Cost Savings: Improved forecasting accuracy leads to optimized inventory management. A study by McKinsey found that companies using advanced analytics can reduce excess inventory by as much as 25%. Take Walmart as an example; they have implemented AI-driven inventory management systems that have helped them reduce stockouts by 30%, which ultimately enhances customer satisfaction and drives sales.
Enhanced Customer Experience: Accurate forecasts ensure that products are available when and where they are needed, which meets customer demands effectively. A retail chain that accurately predicts demand can improve its in-stock rate from 85% to over 95%, significantly increasing customer loyalty and repeat business.
How to Use AI for Forecasting
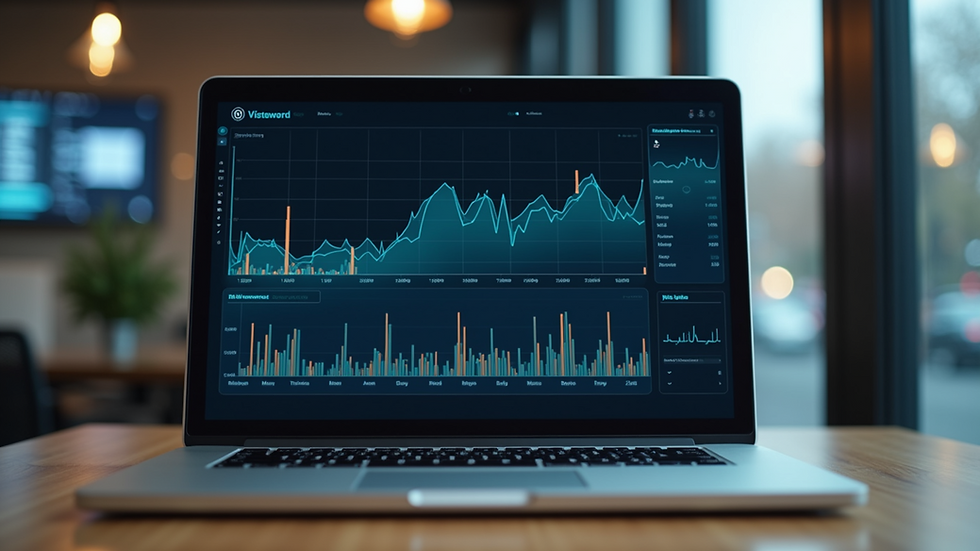
When implementing AI for forecasting, you generally have two main options: utilizing pre-built platforms or developing custom solutions.
Pre-built Platforms
Amazon Forecast: This fully managed service utilizes machine learning to help you forecast future demand effortlessly. Retailers have seen forecast accuracy improvements of up to 50%.
Google Vertex AI AutoML: This platform simplifies the training and application of machine learning models tailored to your specific data needs.
Microsoft Azure Machine Learning AutoML: This tool makes it possible to create predictive models even if you lack extensive data science expertise.
H2O.ai: Renowned for its user-friendly interface, it's a great choice for those new to machine learning.
Custom Solutions
If you prefer to build a unique system, consider diving into programming libraries and data visualization tools:
Python Libraries: Frameworks like TensorFlow and PyTorch are essential for creating custom machine learning models tailored to your forecasting needs.
Data Visualization Tools: Tools like Power BI and Tableau can help present your forecasting insights in a clear, visually appealing way, helping stakeholders understand the data better.
Skills Needed for Successful AI Implementation
To ensure a smooth AI forecasting implementation, you’ll need several key skills:
Platform Familiarity: Understanding your chosen platform, whether pre-built or custom, is essential for effective use.
Data Analysis Skills: A solid grasp of basic data principles will enable you to interpret results correctly. Companies with strong data analysis capabilities are 5 times more likely to make faster decisions than their competitors.
Programming Knowledge (Python): If going the custom route, familiarity with Python is critical for coding and developing your models.
Metrics for Measuring Success

Understanding the impact of your AI forecasting efforts requires tracking specific metrics:
Forecast Accuracy: Use metrics like Mean Absolute Error (MAE) and Mean Absolute Percentage Error (MAPE) to evaluate how closely your forecasts align with actual outcomes. Improving forecast accuracy by even 10% can lead to substantial profit increases.
Operational Efficiency: Look for reductions in time and costs related to inventory management. For instance, businesses can reduce operational costs by as much as 20% with better forecasting.
Revenue Impact: Track improvements in revenue linked to enhanced stock management. Companies that adopt AI forecasting often report revenue growth rates above 10% over standard methods.
Customer Satisfaction: Measure increases in customer satisfaction as you more effectively meet their needs. Satisfied customers are likely to lead to an increase in repeat purchases by up to 30%.
Final Thoughts
AI adoption focuses not just on the latest tech but on solving real business challenges. The AI-powered approach to forecasting transforms how companies make decisions, helping them stay ahead of competitors and enrich customer experiences.
Whether you explore pre-built platforms or build a custom solution, understanding the tools, skills, and metrics involved in AI forecasting is vital for achieving success. As you embark on your AI journey, remember that the real magic lies in how you apply this technology to solve challenges. Seize the opportunities that AI forecasting offers, and watch your business flourish!
Kommentare